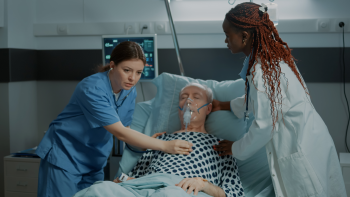
Millions of Americans are infected by influenza annually, but only a fraction of those infected seek care in the emergency department (ED), with even fewer experiencing severe disease or death. A recent study from researchers at Johns Hopkins CEIRR (JH-CEIRR) sought to aid ED clinicians in distinguishing which ED patients infected by influenza can be safely discharged (in need of critical care), and which are at risk for deterioration (in need of inpatient care). The authors used random forest machine learning (ML) models to distinguish between the need for critical care and inpatient care in patients with influenza. Lead author Jeremiah Hinson, M.D., Ph.D., along with research associate Katherine Fenstermacher, Ph.D., and JH-CEIRR principal investigators Andrew Pekosz, Ph.D. and Richard Rothman, M.D., Ph.D., published this work in the Journal of the American College of Emergency Physicians Open.
Deciding which patients that come into the ED with influenza and can be safely discharged is made within hours of presentation and comes with many challenges, including time constraints and limitations on available information. Suboptimal ED disposition decision-making – the process of determining where a patient will transition following the end of their ED visit – leads to increased mortality and excess healthcare expenditures. Electronic health records have been an invaluable source for clinical insight and have been used to train ML algorithms to identify patterns and predict outcomes in various disease states. For example, recent studies1,2 suggest that ML can aid in clinical decision making during all stages of a visit in a SARS-CoV-2 setting. Although influenza has been the leading cause of pandemics in the past and remains a major threat for future pandemics, it has not received the same level of attention in this capacity. As such, the authors’ primary objective was to create and validate a series of ML models to predict severe clinical outcomes in ED patients with influenza.
A retrospective cohort analysis was performed using data from five EDs in the Baltimore area that averaged a combined patient volume of 270,000 ED visits per year. All adults who tested positive for influenza during their ED intake were included in the study. The primary outcomes for the random forest ML models were the need for critical care and inpatient care in patients with influenza within 24 hours and 72 hours of patient disposition, respectively. Predictor data included demographics, ED complaint, medical problems, vital signs, supplemental oxygen use, and laboratory results.
Model performance was measured using receiver operating characteristic (ROC) and the Brier score. The Brier score is an estimate of accuracy, 0-1 with 0 representing perfect accuracy. Area area under the ROC curve is a summary metric of the ROC which reflects the test’s ability to distinguish between outcomes with a scale of 0-1. A score of 0.5 indicates random guessing and a score of 1 indicates perfect performance. The results showed that there was a 6.3% incidence of critical care needs and a 19.6% incidence of inpatient care needs. Model performance was assessed to be 0.89 (95% CI 0.86-0.93) for prediction of critical care and 0.90 (95% CI 0.88-0.93) for prediction of inpatient care, with Brier scores of 0.026 and 0.024, respectively. Shortness of breath, increased respiratory rate, and high number of comorbidities were the strongest predictors.
These results suggest that ML methods can be used to accurately predict clinical deterioration in ED patients diagnosed with influenza and may support ED disposition decision-making in the future. However, optimal use requires the ability to incorporate real-time electronic health record data processing and the output of a specific and actionable care decision, which is a future goal for the research team. This study will serve as a basis for the development, implementation, and maintenance of ML clinical decision support to optimize ED care for patients with influenza. The authors aim to reduce mortality and lower healthcare expenditures.
To learn more about how the authors developed and implemented the ML model, read their publication: Hinson J. et al. (2022) Multisite development and validation of machine learning models to predict severe outcomes and guide decision-making for emergency department patients with influenza. J Am Coll Emerg Physicians Open. 5(2): e13177
To learn more about how ML algorithms are being used to help treat patients with SARS-CoV-2 check out:
- Wynants et el. (2020) Prediction models for diagnosis and prognosis of covid-19: systematic review and critical appraisal. BMJ. 369: m1328
- Hinson et al. (2022) Multisite implementation of a workflow-integrated machine learning system to optimize COVID-19 hospital admission decisions. NPJ Digit Med. 5:94